Healthcare AI: The Importance of Hospitals and Health Systems Focusing on AI Governance
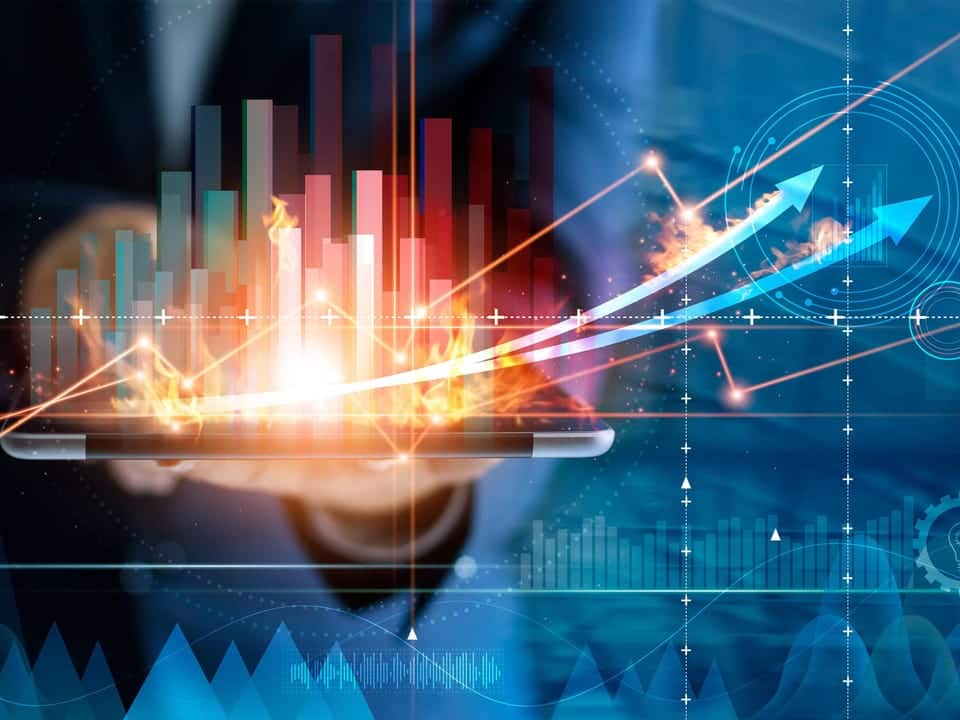
EHR and third-party vendors have made AI solutions and models commercially available, enabling artificial intelligence (AI) in healthcare to reach a turning point in adoption and usage. However, most health systems have not developed an enterprise strategy for how AI should be introduced, invested in, and managed within their organization. This leads to a lack of trust in AI-powered solutions. Senior analytics leaders need to take a lead role in ensuring there is discipline and accountability around the use of AI in health systems.
Automated AI Products are Now Commercially Available
Artificial intelligence (AI) in health delivery has long suffered from years of build-up and unrealistic expectations. Although the hype surrounding AI persists to a certain degree, there is no denying that the health delivery industry is at a genuine turning point with automated AI products. As EHR vendors and tech giants have steadily gained traction in the AI space over the last 2-3 years, the barrier to entry for hospitals and health systems has been dramatically lowered. AI models and algorithms that previously had to be developed and maintained “in-house” are now commercially available. Vendors of these products assume responsibility for the maintenance of the algorithms in their AI tools, thereby eliminating the need for a hospital or health system to employ a dedicated team of data scientists to develop and support custom AI models. However, algorithms within commercially available AI products can be trained using the data of a specific organization, ensuring that the AI models deployed are tailored to a hospital or health system’s unique patient population.
Although the platforms and tools needed for success with AI are now far more accessible to the average health system, that alone doesn’t translate into realizing value. Hospitals and health systems still need to focus on establishing and optimizing the governance of AI.
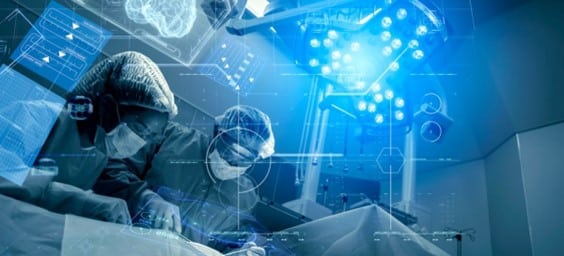
Governance of AI Is Essential
The integrity and quality of the underlying data upon which AI models are trained is critical to ensure accuracy and remove the risk of model bias and transparency. To drive successful AI initiatives at scale, healthcare organizations will require a comprehensive AI governance strategy as a foundational pillar.
There may not always be much context or transparency as to how an automated AI product reaches a given conclusion. For example, a commercially available predictive AI model focused on readmissions may assign a patient a specific score indicating their level of risk to be readmitted, but may provide little else in the way of details, such as the accuracy and reproducibility of the model. This relative lack of transparency – especially given the ethical issues and regulatory requirements associated with AI solutions – is one of the primary reasons why it is so critical that health systems have the right governance in place. This governance can enable continuous monitoring and auditing of automated AI solution performance, as well as support efforts to bridge the gap between “technical speak” and “plain English” to ensure key stakeholders are fully empowered to make informed decisions.
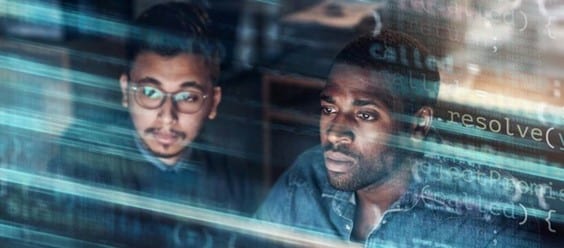
The right AI governance is vital from the start, as the models in any automated AI product need to be trained on the specific patient population of the hospital or health system where the solution will be deployed. After being trained, the provider organization must do its due diligence to ensure the model is performing equally well across a range of new clinical settings and, if not, determine whether the algorithm can be retuned or recalibrated using local data to account for differences in population characteristics or care protocols. (For example, a model’s performance could initially be affected if a health system’s patient population is significantly different than the population on which that model was originally built.)
Keys to Success with AI Governance
Approaches and strategies will vary depending on a variety of factors – the size and complexity of a given hospital or health system, the provider’s overall goals and unique strategic priorities, etc. – but there are still several key tenets for successful AI governance that apply to any organization:
- Ensuring executive sponsorship
- Clearly defining the objectives and purpose of an AI solution
- Assessing the suitability of deploying an AI solution
- Implementing the right internal governance structures and measures
- Determining the level of human involvement in AI-augmented decision-making
- Actively interacting and communicating with stakeholders
- Clearly defining roles and responsibilities
- Developing a formal AI communication plan
- Establishing defined performance targets for AI models
- Ensuring alignment between automated AI solutions and the strategic priorities of operational business owners
- Conducting regular audits to assess performance, compliance, and consistency of AI models
- Focusing on “storytelling” and “going the last mile” to affect patient outcomes
Measuring performance is not a one-time initiative. The models within automated AI products must be regularly assessed and audited to ensure they continue to work as intended. In many cases, after a commercially available AI tool is trained and deployed, it may simply be automated and then largely left alone. It is important to remember that an “automated” solution is very different from an “immutable” or “infallible” one. Every AI model will experience “drift” over time as environmental factors, workflows, and countless other variables change. As such, hospitals and health systems need to be able to understand – and effectively communicate to key decision-makers – how a given model is supposed to work and how it is performing in the current environment.
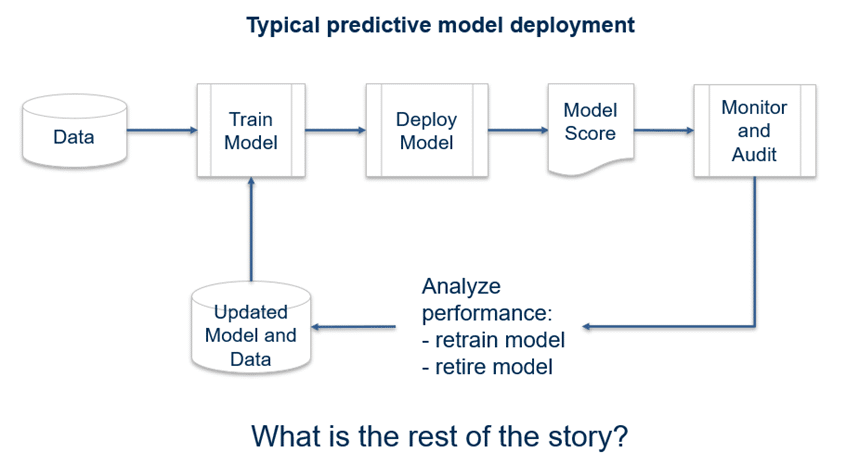
One of the most important things a senior analytics leader can do is to tell the “rest of the story.” AI is like running a marathon, and just like the race is not over until the last mile, the AI model is not complete until the rest of the story is told. This “last mile” with AI is where staff take the stories the data is telling and connect it to themselves and their patients, such as tying a strategic KPI to an opportunity analysis to identify the care that needs to change. If a hospital identifies reducing the number of sepsis deaths as a priority, timely intervention to prevent complications from sepsis needs to occur in the ER, so an optimized workflow should start with the ER team.
Impact Advisors’ AI Audit Framework – How We Can Help
Artificial intelligence systems for healthcare, like any other medical device, have the potential to fail. However, specific qualities of artificial intelligence systems – such as the tendency to learn spurious correlations in training data, poor generalizability to new deployment settings, and a paucity of reliable “explainability” mechanisms – mean they can yield unpredictable errors that might be entirely missed without proactive investigation.
Impact Advisors’ algorithmic audit framework is a tool that can be used to better understand the weaknesses of an artificial intelligence system and puts in place mechanisms to mitigate their impact. We provide an algorithmic audit framework that guides the auditor through a process of considering potential algorithmic errors in the context of a clinical task, mapping the components that might contribute to the occurrence of errors, and anticipating their potential consequences. We suggest several approaches for testing algorithmic errors, including exploratory error analysis, subgroup testing, and adversarial testing, and provide examples from our work and previous studies. Safety monitoring and medical algorithmic auditing should be a joint responsibility between users and developers. Encouraging the use of feedback mechanisms between these groups promotes learning and maintains the safe deployment of artificial intelligence systems.
Contributor: Victor Collins, Advanced Intelligence Practice Lead