Harnessing Generative AI in Healthcare: A Pragmatic Guide to Accelerate Impact
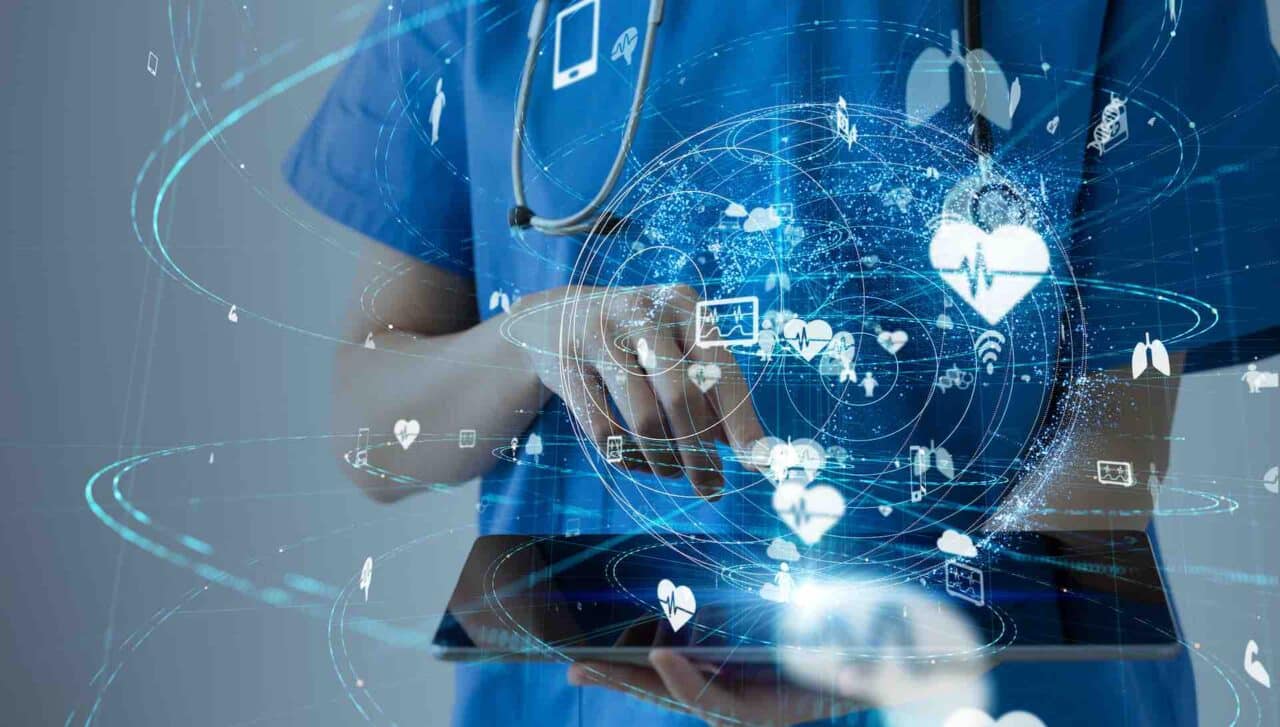
With the healthcare industry facing a perfect storm of challenges, including an acute staff shortage, escalating clinical costs, and declining reimbursement rates, the burgeoning field of Generative (Gen) AI offers a beacon of hope.
Healthcare has been slower to embrace AI than other industries, but Gen AI is poised to change that significantly. It holds the promise of improving healthcare quality, efficiency, and accessibility by automating and enhancing various medical tasks, including those related to imaging, diagnosis, and patient care.
What is Gen AI?
Gen AI represents a significant advancement in the field of artificial intelligence and fundamentally changes how business processes are designed, executed, and optimized. While machine learning (ML), natural language processing (NLP), and computer vision (CV) have been used in healthcare over the past decade, those instances have been limited and, in many cases, have represented expensive investments that did not always result in lasting value or transformative change for many early adopters.
What is new – and what has been the primary catalyst for the recent explosion of interest in Gen AI – are the large language models (LLMs) that emerged in the early 2020s. LLMs have proven to be exceptional at understanding human writing, speech, and images and represent a subset of AI capable of generating human-like logic and output based on massive training datasets. LLMs, trained on publicly available data, debuted in December 2022 with the release of ChatGPT, which became the fastest-growing consumer technology application ever to reach 100M users in six weeks. For the first time in history, humans can interact with computers as they would another human.
Focus on Business Domains to Move Forward
In healthcare, Gen AI has the potential to fundamentally change existing processes and workflows. We see four areas where Gen AI can drive meaningful incremental value.
-
Content Generation and Personalization:
Develop personalized communications to providers, payors, regulators, and patients, leveraging vast amounts of information to create simple summaries in near real-time, as well as providing content for individual care and treatment.
-
Patient Engagement:
Support the engagement of patients during onboarding and post-discharge in areas such as medication adherence, summarizing health plan benefits and post-discharge summaries.
-
Virtual Health Assistant/Co-pilot:
Provide 24/7 support to nurses, care managers, and other healthcare professionals by automating routine tasks such as prior authorization, and augmenting more difficult ones, such as optimizing referrals, thereby dramatically increasing staff productivity.
-
Clinical Diagnosis and Imaging:
Enhance medical imaging interpretation, automate part of the process, and reduce the burden on radiologists, thereby supporting the clinical diagnosis process for providers.
From secondary research and our client experience with provider organizations, the chart below illustrates where we believe the biggest economic lift will come from the adoption of Gen AI in terms of the productivity benefits by business domain (i.e., the benefit opportunities will accrue heterogeneously across business domains).
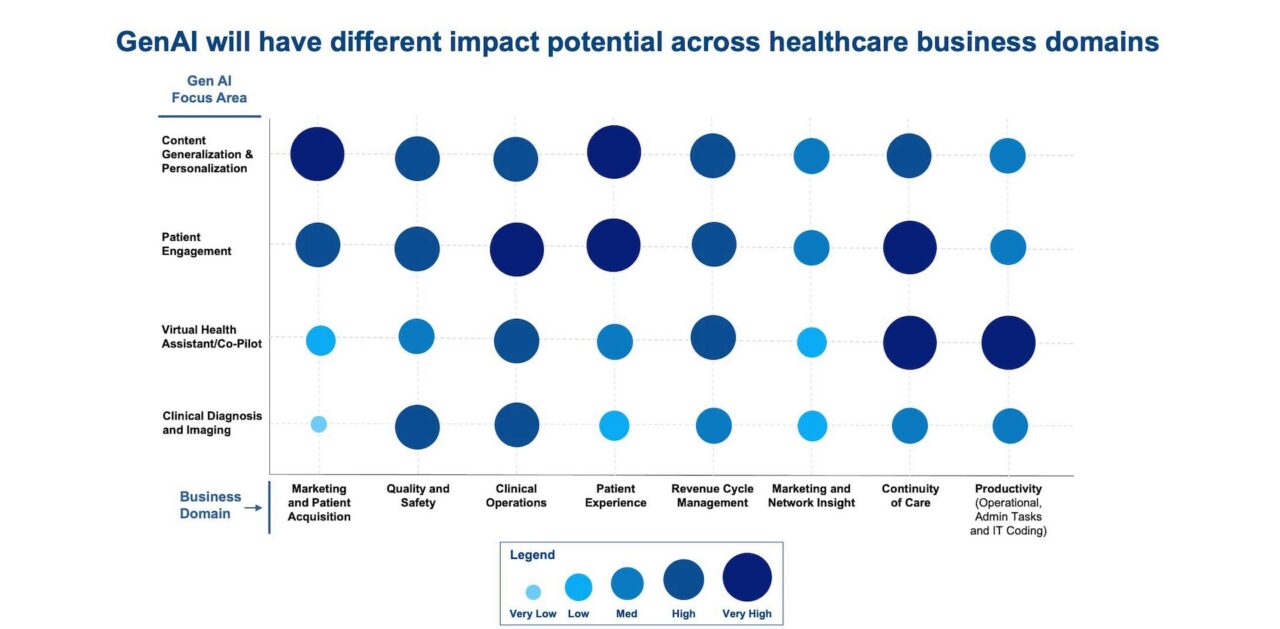
Figure 1
Let’s examine two domains in particular where there is evidence from several provider organizations that are already seeing the benefits of applying Gen AI.
Clinical Operations
Clinical operations is dedicated to ensuring that healthcare delivery is efficient, effective, and patient-focused, leading to optimal health outcomes. Traditional AI has the ability to analyze historical patient data – and predict and prescribe personalized next-best actions at any point in the care journey. Traditional AI also stratifies patients by risk, predicting the likelihood of readmissions and handling transitions of care. Gen AI is transforming this domain by analyzing real-time patient data to generate a complete end-to-end care journey, including language and documentation for other caregivers, physicians, and family members. Gen AI also has the ability to continuously learn and adapt to optimize care coordination dynamically, enhancing communication and responsiveness to patient needs.
NYU Langone Health has demonstrated the advantages of Gen AI over traditional machine learning models in predicting patient readmissions, hospital stay durations, and mortality risks. Their AI program, which interprets physicians’ notes, is currently in use at NYU Langone Health hospitals to forecast the likelihood of patient readmission within 30 days. A study published by NYU Grossman School of Medicine in the journal Nature introduced an LLM named NYUTron, which trained on raw text from health records and outperformed standard models by predicting readmissions with an 80% accuracy rate – a 5% improvement over older models.
Claims Management and Revenue Cycle
Claims management is a crucial part of healthcare operations, involving the submission and tracking of insurance claims to ensure providers receive payment for their services. This ties into the broader scope of revenue cycle management, which encompasses all the financial processes of a healthcare practice, including patient scheduling, billing, and collections. Traditional AI streamlines and accelerates the process of accurate and compliant claims submission, reducing manual errors and ensuring timely submissions to payors by enhancing the assignment of correct medical codes and charges, minimizing errors, and optimizing revenue through accurate billing. Gen AI is uniquely capable of automating lower-value submissions and clinical audits – and synthesizing disparate data sources, payor requirements and policy changes. It also generates more accurate real-time coding recommendations, modifiers, and draft documentation based on medical records.
Implementing Gen AI co-pilots in this space allows organizations to fully automate the most labor-intensive tasks around submissions and audits and provide enhanced real-time analysis for revenue cycle professionals for the most complicated and highest-value scenarios. Banner Health slashed administrative burden and boosted efficiency by automating insurance coverage discovery. They achieved this through a smart combination: a service that automatically identifies a patient’s coverage and a bot that seamlessly updates it across their financial systems.
A Practical Framework for Gen AI Transformation
There are five key elements to a successful Gen AI transformation, each with unique considerations that need to be addressed.
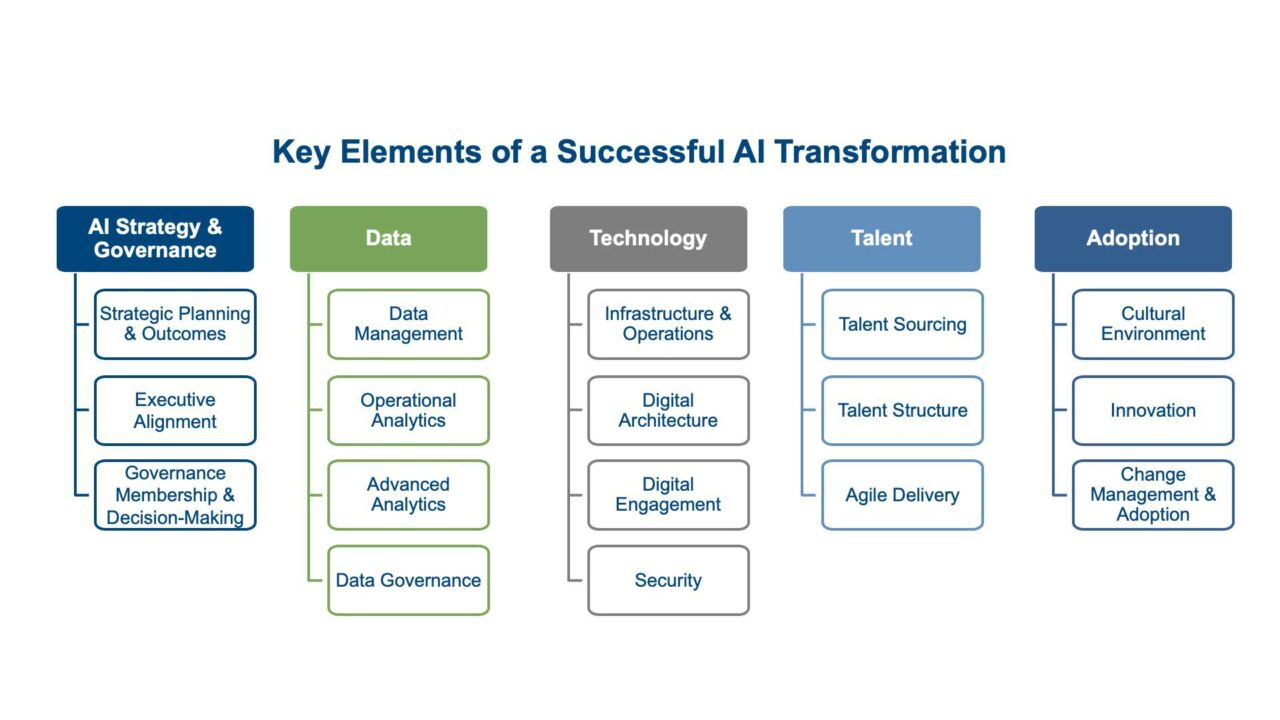
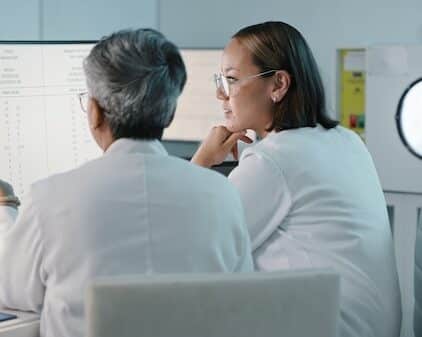
AI Strategy and Governance – Unique Challenges
Despite the success stories highlighted above, many hospitals and health systems are struggling to realize Gen AI’s potential to completely transform and reimagine the way they do business. Per a recent survey from Bain & Company, while “75% of health system executives believe Gen AI has reached a turning point in its ability to reshape the industry… only 6% have an established Gen AI strategy.” Applying Gen AI to a long list of discrete use cases is an approach that is unlikely to produce consequential change. Yet trying to overhaul the whole organization at once with Gen AI is simply too complicated to be practical.
We suggest starting with two or three business domains in parallel that are feasible to execute (e.g., Clinical Operations and Claims Management and Revenue Cycle, as highlighted above) to determine the value of Gen AI to your organization. From there, apply the lessons learned and the organizational momentum gained to then tackle the next highest priority domains over 12 to 18 months.
From our client experience, an integrated Gen AI strategy to transform business domains has the potential to drive a 15% to 40% improvement in bottom-line economic results for a provider as illustrated below in Figure 2. This is a 20% to 60% lift relative to the improvement from adopting traditional approaches to AI.
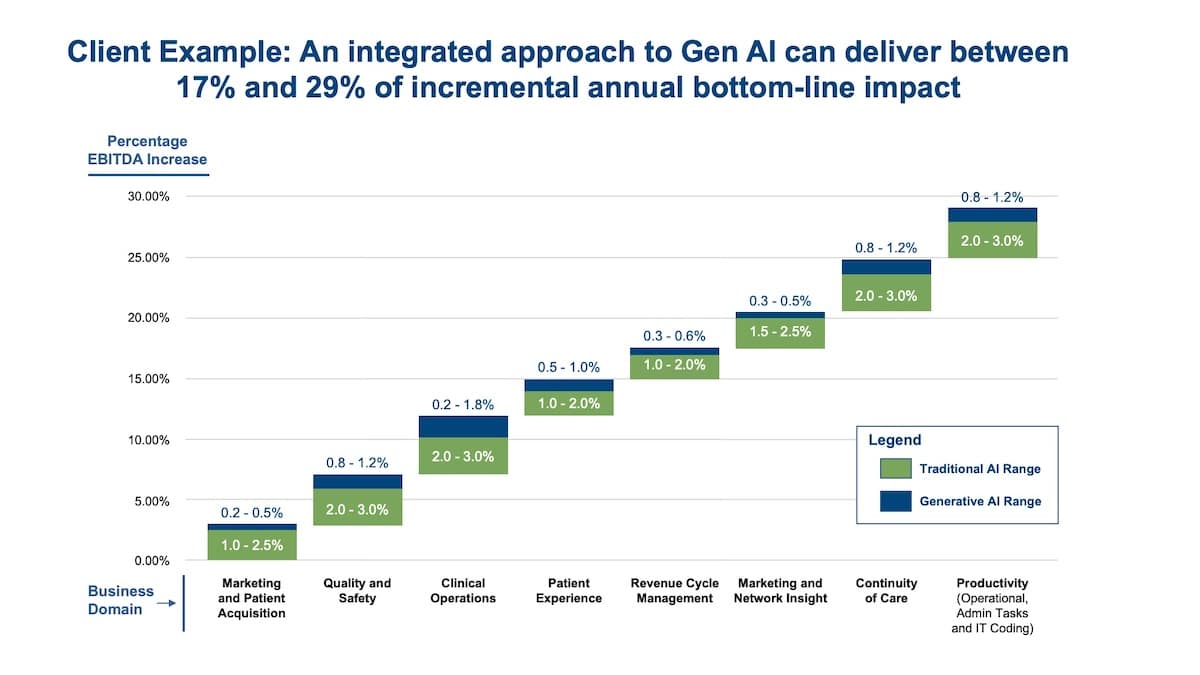
Figure 2
Even though there are significant opportunities by applying Gen AI, there are also risks that need to be acknowledged and mitigated. The governance of Gen AI presents unique challenges related to the production of synthetic data, intellectual property usage rights, ethics, and privacy to name a few. Gen AI is a potentially transformative technology in healthcare that needs to be managed carefully by balancing the need for innovation with the appropriate guardrails that protect patient data and overall health outcomes.
The Seven Dimensions of LLM Trustworthiness is a good framework to consider when developing your Gen AI governance approach. This question of LLM Alignment refers to making models behave in accordance with human intentions. The Seven Dimensions of LLM Trustworthiness ensure that LLMs consistently provide accurate and reliable information, prevent harm, eliminate bias, resist misuse, operate transparently, uphold societal values, and remain robust against cyberattacks or data changes. These principles are fundamental to maintaining trust as LLMs become more prevalent in healthcare applications.
Some specific governance examples include:
- Clinical Trials – AI’s potential to supplant human subjects demands rigorous validation of data integrity and trial outcomes.
- Personalized Medicine – Governance mandates the validation of AI-crafted treatments’ safety and equitable distribution.
- Medical Training – The fidelity and impartiality of AI-synthesized case studies are non-negotiable.
- Medical Imaging – Stringent controls are essential to prevent the misapplication or erroneous record integration of AI-generated images.
Impact Advisors has developed a playbook to give you the practical guidance for how to apply sophisticated governance protocols to your Gen AI program.
Data – The Differentiator in Gen AI
As the development of LLMs mature, AI teams have proven that training on vast amounts of industry-specific data yields more accurate predictions and content than the generic models that have been trained on the publicly available data on the internet (e.g., ChatGPT). AI companies investing in gathering more specialized healthcare data like clinical feeds, clinical trial data, prescriptions patterns and outcomes, health plan policies, and medical exams to train healthcare-specific models are outperforming the non-industry specific LLMs. Models like Med-PaLM 2, released by Google in January 2023, are trained on datasets like the Cochrane Library, clinicaltrials.gov, Harrison’s Principles of Internal Medicine, and MedQA so they can specifically answer detailed medical questions. These models are then tested and measured on a full suite of medical exams to ensure their responses can be judged as if they were provided by a healthcare professional.
The initial building and training of these models historically required significant investment and proprietary Intellectual Property (IP) and could only be done by large or dedicated technology organizations. However, due to the open-source community and some AI service organizations like AWS, Hugging Face, etc., large healthcare organizations can train and heavily tune LLMs directly on their own proprietary data. CIOs need to strike the right balance between integrating off-the-shelf healthcare LLMs and exploring the possibility of investing in the creation of their own IP.
Taking a phased approach will lead to the best results. After selecting the top priority business domain(s), the next step is to select the right partner with a mature solution that can prove the value. Once your organization can track the value end-to-end, you can look to use proprietary data as a differentiator in the creation of new custom LLMs specific to your organization and patient populations.
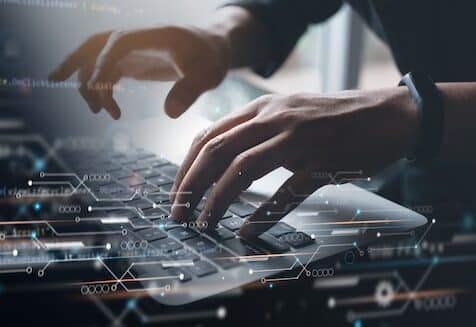
Technology – Gen AI Models / Systems Are More Complex
In the past, AI researchers and engineers used central processing units (CPUs) to run rule-based systems and basic algorithms. But as AI has evolved, graphics processing units (GPUs), tensor processing units (TPUs), and specialized neural processing units (NPUs) have become essential for running large neural network models (e.g., deep learning). Gen AI models are particularly demanding of hardware resources, requiring large amounts of data and computational power.
Cloud platforms like AWS, GCP, and Azure now offer access to high-performance computing resources on demand. This has made it possible for researchers and businesses to develop and deploy Gen AI models without having to invest in their own infrastructure.
Another key shift is the move from simple decision trees and linear regressions to massive neural networks to target and achieve the benefit lifts outlined above. These neural networks are trained on large datasets to learn complex patterns and relationships. This has enabled AI models to perform tasks that were previously impossible, such as giving personalized patient recommendations and interventions based on all the information known about the patient or suggesting the newest leading clinical procedure recommendations based on the most recent knowledge shared across the entire medical community.
Organizations that want to leverage these complex AI technologies should first adopt solutions from outside partners who can bear the burden of hosting and deploying the AI infrastructure while easily integrating within existing workflows. This allows healthcare organizations to focus on adopting clinical and patient use cases to ensure the highest chance of adoption and return on investment. This highlights a huge opportunity for the large EHR vendors and the solutions that tightly integrate with them.
As your organization develops a successful track record and “muscle memory” for deploying AI solutions, you can then begin to assess the pros and cons of supporting the tech stacks, data, and talent needed to host and fine-tune custom LLM solutions and the associated business benefits of deploying these more advanced capabilities.
Talent – Technical Workforce Changes to Develop Gen AI Solutions
As organizations pivot towards AI-driven solutions, there is a shift from traditional IT roles to more specialized AI roles. This means a greater emphasis on hiring and training for positions such as Machine Learning Engineers, Data Scientists, Data Engineers, Cloud and DevOps Engineers, User Experience (UX) Designers to ensure the last mile integration of these AI solutions, and Prompt Engineers who own the technical interaction, implementation, output reliability, and workflow of Gen AI solutions.
These roles are pivotal in the development and deployment of Gen AI in healthcare. Their expertise is crucial in adapting LLMs to specific tasks or domains, and ensuring the technology is integrated into existing architecture and user workflows. Having UX Designers map out the ideal end-to-end workflow, for example, after a Gen AI solution summarizes a physician’s note, will ensure the highest chance of adoption. This will identify points in the process that need human in-the-loop intervention and highlight areas where Machine Learning Engineers can further automate the AI pipeline around that specific use case.
Gen AI models are data-hungry, so Data Engineers are also essential for this field. They are responsible for creating robust data pipelines to ensure that high-quality data is available for model training. Once the model is trained, Cloud and DevOps Engineers are responsible for deploying it to production and ensuring it is integrated seamlessly with existing healthcare systems. This requires a deep understanding of both the model and the target system.
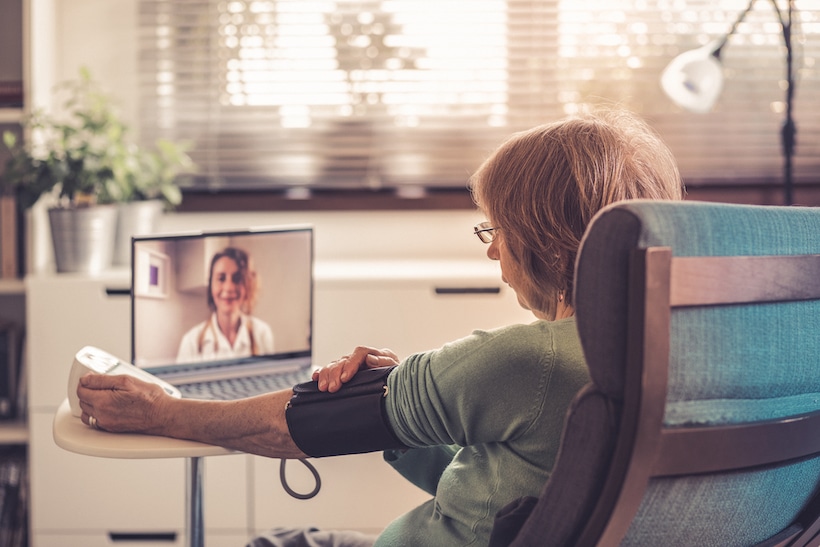
Adoption – Evangelizing the Benefits of Gen AI
The level of sophistication of an AI model is irrelevant unless it results in a human making a better decision (augmented by the machine) that leads to a preferred clinical or business outcome. This is what we call the “last mile” of AI – which is adoption – and is typically the most challenging to get right.
Adoption in clinical areas should take a risk-based approach focusing on the impact to patient outcomes. One should think of the risk of a “bad” decision in deploying these models, and, as such, adoption in non-clinical areas (e.g., automated population of forms with appropriate codes for claims submission) is likely to happen more easily than in clinical areas (e.g., recommendations around medication changes for a patient with multiple chronic conditions). As an AI colleague of ours recently observed about moving from the technology sector to healthcare, “There is a far greater impact from developing AI to support a decision that impacts a person’s health than a marketing decision at a big tech company.” For this reason, the adoption of AI in healthcare has been slower than other industries, since the closer you are to the clinical decision, the higher the risk of making a bad, potentially fatal, decision.
We suggest taking a “last mile” first approach. After you determine your business domain focus, identify which decisions and roles will be changed by the introduction of the new AI capability. The integration of AI into business processes requires close collaboration between domain experts and technical teams. This might lead to the emergence of roles like AI translators or liaisons who bridge the gap between business stakeholders and AI experts.
Gen AI will significantly alter the jobs of healthcare workers, such as physicians, nurses, and administrative staff. Think through which roles are impacted and what potential insights will be available from Gen AI to augment the knowledge of the person in the role. For example, in the case of a specialty care nurse providing chronic care to a patient remotely, the AI can summarize the chronic conditions to check for, suggest the next best question to ask the patient based on their recent clinical and contact history, and then summarize the patient progress note. This is opposed to fully automating the patient care decision-making process, which we believe in this case would not only be unrealistic, but irresponsible and negligible.
The next step is to think through how the AI insights are integrated into the workflow in order to make it easy to adopt in a clinical setting, such as at the point of care. For example, in the case above, the Gen AI model produces the post-discharge care summary for the patient, while the specialty care nurse emphasizes the key points regarding transition of care, medication adherence, and specialist follow-up appointments.
Anticipate the barriers to the effective deployment of this change and navigate them accordingly. For example, the specialty care nurse could feel threatened that Gen AI will make his or her role obsolete. Determine how to best engage the nurse through communication, training, incentives, or recognition to diffuse the threat. By proactively stressing the benefit of Gen AI to automate some portions of the job, the specialty care nurse can focus on more value-added activities.
Build the organizational “muscle” to make AI and Gen AI adoption a key differentiating capability for the enterprise. This starts by building momentum from the success of the first few domains that are transformed so that others in the organization can see how it is done. It includes broad scale awareness and education about how Gen AI can transform the way work is performed and enables overburdened clinicians to focus more of their time with patients.
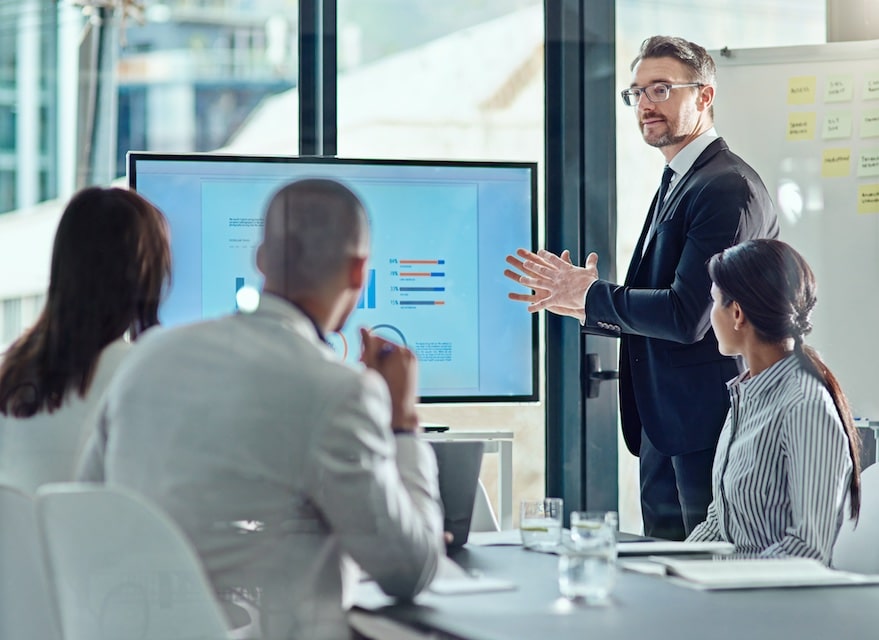
“Last year AI was truly an out-of-body experience for the organization. This year it is real and is impacting us now - and in the next year or so it needs to become part of our cultural DNA.”
How to Get Started in the Next 90 Days
- Determine the business domains, such as Clinical Operations or Claims Management and Revenue Cycle, that have the highest potential impact from Gen AI adoption and are most feasible to get to minimum viable products (MVPs) within three months.
- In parallel, assess your data environment to determine what is unique and proprietary versus broadly accessible. In terms of the technology stack and talent enablers, determine how to work with partners to acquire these capabilities rather than through internal investment.
- Start by targeting two to three domain MVPs to target to prove the value and test out the operating model in parallel. For example, one provider chose to start in clinical operations focusing on the ability to analyze real-time patient data to generate a complete end-to-end care journey, including language and documentation for other caregivers, physicians, and family members. Another hospital system focused on prior authorization with a live co-pilot that synthesizes disparate, unstructured data to generate nuanced evaluations and recommendations, allowing for more precise and personalized approval decisions.
By starting down this path now, in the next 90 days you will be significantly further along than most provider organizations, as the level of change that Gen AI enables in transforming healthcare processes is unprecedented.
Contributors:
Liam Bouchier, Vice President, Data & AI, Impact Advisors
Joe Christman, Vice President, AI & Digital Platforms, Chicago Pacific Founders
Victor Collins, Associate Director, Data & AI, Impact Advisors
Brian McCarthy, Board member of Impact Advisors and Operating Partner, AI & Digital Platforms, Chicago Pacific Founders